All charts by Emir Cuk
Capture and use digital data correctly
The analysis of a production starts with the bottlenecks. This prevents production from being optimized locally at the points that contribute little to the performance of the entire production system.
Below we present four case-specific methods and tools from our daily production optimization practice. It is important that the analysis effort is in reasonable relation to the complexity of the production optimization problem in order to choose the right approach.
Fill bottlenecks with value chain analysis
Value stream analysis provides a complete overview of production, including planning and control. The overall process is visible to everyone. We can identify critical processes based on value stream-specific key figures.
We perform the analyses on-site with paper and pencil. That makes for quick analysis. You get an impression of order and cleanliness as well as actual on-site conditions on the shop floor. You can talk to employees and benefit from their knowledge.
Results can be used immediately and easily communicated, thanks to transparent presentation. The situation depicted in the value stream analysis, however, only represents one point in time. Processes with high utilization – potential bottlenecks – can be easily identified using the key figures that are collected. These can then be precisely analyzed with an appropriate tool: OEE app for simple systems, LeanDA for manual processes, and MOEE for complex, highly automated processes.
Understand bottlenecks’ causes with the OEE app
In multi-step manufacturing, it is important that we understand bottlenecks’ dynamic behaviors. Workers who operate one or multiple machines can digitally record system performance with little effort, and evaluate them immediately. The worker can record status transitions simply using a touch tablet. The subsequent evaluation assesses overall system effectiveness (Overall Equipment Effectiveness, or OEE) of the plants, and provides conclusions about the causes of bottleneck behavior.
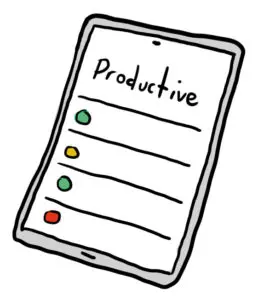
A great advantage of this simple tool is low-effort application, and extremely fast results generation. It only takes a about half an hour to define and enter the machine states of the selected system in advance. We have first data and analyses already after the first shift. The OEE app has already been successfully used in several projects. Additionally it supports the reevaluation of plan times.
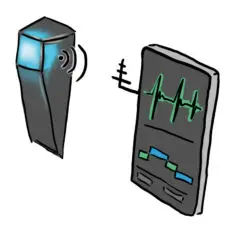
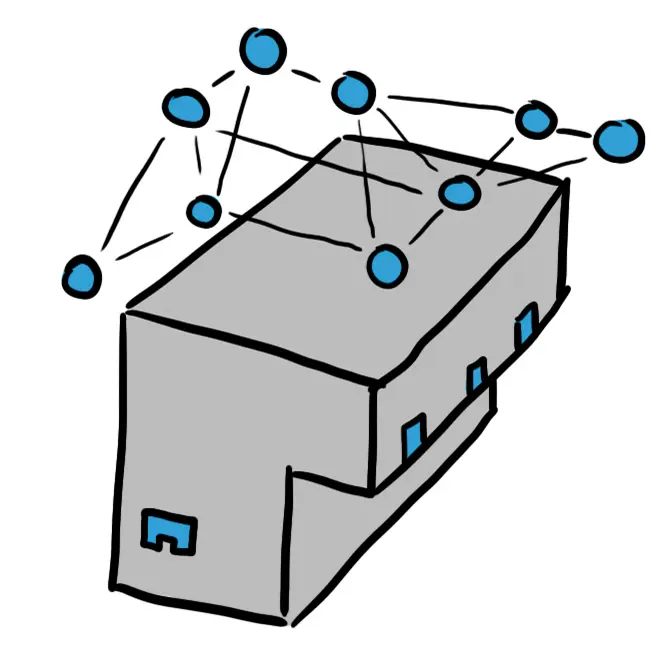
LeanDA for root cause analysis in manual processes
In order to have both hands free for value-adding activities in manual assembly, we are replacing the touchscreen with wireless sensors that automatically record movement data. Similar to the OEE app, process steps are predefined, and sensors attached to tools and components. The pre-filtered acceleration, rotation and magnetic field signals for all three axes serve as input signals for process recognition through machine learning. After a short learning phase using a tablet, the algorithm automatically recognizes the processes being executed, providing process engineers with the key performance indicators required to evaluate processes. This systematic transformation of signal data (big data) into information (smart data) enables process engineers to find clues for process optimization. This solution, called LeanDA , has already been applied in industrial projects and can be experienced in the Future Work Lab of Fraunhofer IAO and IPA in Stuttgart.
MOEE: Intelligent production line optimization tool for root cause analysis within interlinked systems
We have developed a software tool with MOEE that offers the ability to automatically identify the causes of productivity losses in machines and interlinked manufacturing lines. We use algorithms to thoroughly examine complex, interlinked systems and uncover losses related to performance, availability and quality.
More about LeanDA
- to the LeanDA product information sheet
- to the page about LeanDA on the Fraunhofer IPA website
- Article from the magazine “Automationspraxis” about the use of LeanDA at Elabo: »Sensorik hilft bei der Montageoptimierung«
We recognize dynamic bottlenecks at the time that they occur, thus, contributing to the rapid elimination of errors, using a combination of automatic process model creation and machine learning algorithms. The additional drill-down function breaks down processes into sub-steps and shows critical process steps. Root cause analysis identifies the causes of errors down to the component level. By shortening the troubleshooting process, the maintenance technician can directly focus on repairing the identified component. Either the I/O signals from the machine controller (e.g. PLC) or our own external sensors, which are attached to critical positions in the system as a retrofit solution, provide input data. Additionally we attach cameras to generate additional information. We then merge video images with control signals in order to obtain complete process transparency related to the causes of malfunctions and short downtime.
Therefore this evaluation only requires access to machine controller. The data are evaluated on-site in the company without cloud risks. The system gives us transparency about productivity losses, especially when ramping up new lines, or switch to new products. It can therefore quickly increase productivity. The system has already proven itself with our customers in the cost-sensitive automotive industry and with complex, highly-automated systems in the pharmaceutical industry.
Conclusion
Our consulting approach uses value chain analysis to provide an initial overview and identify bottlenecks. Different tools, depending on technology, are used for detailed root cause analysis. The dynamic behavior of simple processes can be precisely recorded using the OEE app. If more precise analyses are needed, LeanDA supports manual processes with data acquisition, and MOEE supports problems with complex, interlinked systems. Our methods knowledge, combined with digital tools, makes it possible to find the right information in a flood of diverse data.
Your contact persons
Florian Grabi
Member of the research team Data-driven production optimization
Phone: +49 711 970-1925
Julian Maier
Member of the research team Digitized Order Processing
Phone: +49 711 970-1958
Roman Ungern-Sternberg
Head of End-to-End Operations
Phone: +49 711 970-1976