Source: Experian
AI under control
Products that use artificial intelligence (AI) must be developed in accordance with the law and deliver comprehensible results. An audit conducted by Fraunhofer IPA has now confirmed these properties for a product from Experian – the “Fraud Miner” as part of a fraud prevention solution.
Published on 27-10-2022
Reading time approx. 12 minutes
AI in data analysis opens up new possibilities in many industries. At the same time, the technology can be unsettling and raise ethically relevant questions. Particular focus is placed on machine learning (ML) and the algorithms used in this process. As ML systems learn largely independently based on data and the interdependencies in the resulting models are not clear for many algorithms, we often refer to a “black box”, because it is difficult to understand how an algorithm obtains a specific result.
This can become legally problematic, because AI will be subject to more stringent regulations by national and European projects, and it diminishes confidence in the technology. To improve this, objective methods can ensure that ML systems work fairly, and in a clear and comprehensible process. This is demonstrated by a project of Fraunhofer IPA with the information service provider Experian. The Institute examined its ML component “Fraud Miner” of a data processing product for online trading with respect to a legally compliant development and the traceability of the results. The methodology used is applicable to almost all industries and AI-based products.
Machine learning in fraud prevention
The Fraud Miner aims to reliably detect attempts at online fraud. Systems used to date generally detect too many fraud attempts, which in the end turn out to be “false positives”, i.e. falsely declared as fraud. According to the payment consultancy CMSPI, in 2020 the loss of sales due to actual card fraud in Europe was approxately €2 billion, while the losses due to false positives and the manual checks required as a result were approxately €23 billion.
Experian has therefore developed the fraud prevention solution Aidrian in Germany, whose machine-learning component, the Fraud Miner, is designed to detect fraud more reliably and thus significantly reduce the number of false positives. Experian aimed to subject the Fraud Miner to an independent audit to ensure that the solution complied with the respective legislation and delivered comprehensible results. The company approached Fraunhofer IPA with this request in 2021.
How is this tested?
There are still no precise specifications to test an ML system or even any official standards. The most important initiatives for the development of a binding set of rules were proposed by the TÜV (technical certification body) in Austria, the Fraunhofer Institute for Intelligent Analysis and Information Systems IAIS and the European Commission. The latter is currently attempting to establish guidelines for the development of AI applications with its draft for an “Artificial Intelligence Act”. However, these are often too abstract and contain few practical requirements for companies and skilled workers.
TÜV Austria and the University of Linz, presented initiative for the certification of ML systems in a white paper. An essential part is guidelines for auditing ML systems, which incorporates existing criteria from software development and also addresses ethical issues. While the guidelines may be restricted to problem areas, such as supervised learning and largely non-critical applications, it lays the foundation for further certification procedures.
Currently, the most detailed proposal for auditing an ML system is the “Leitfaden zur Gestaltung vertrauenswürdiger Künstlicher Intelligenz” (guidelines for the design of trustworthy artificial intelligence) from the Fraunhofer IAIS. Among other, it contains guidelines for the structured identification of AI-specific risks with regard to the six dimensions of trustworthiness: Fairness, autonomy and control, transparency, reliability, security and data protection. It also provides guidance on the structured documentation of technical and organisational measures along the lifecycle of an AI application that reflect the current state-of-the-art. All three of these works provided important guidance to audit the Fraud Miner.
Technical explainability
Technical procedures can ascertain whether a system such as the Fraud Miner makes comprehensible and fair decisions. For example, the use of “surrogate models”, a simulation of the original model, has proved to be successful. Another method consists of counterfactual explanations, which demonstrate how the results depend on the input data. The SHAP approach (Shapley Additive exPlanations) should also be mentioned, which implements concepts from game theory. This is used, for example, to explain the prediction of a certain value by calculating a correctly-assessed contribution to the prediction of each feature. It is also advisable to use two perspectives around explainability: the global explainability of a model to represent the main drivers and cause-effect relationships, and the local explainability that leads to the outcome in a particular case.
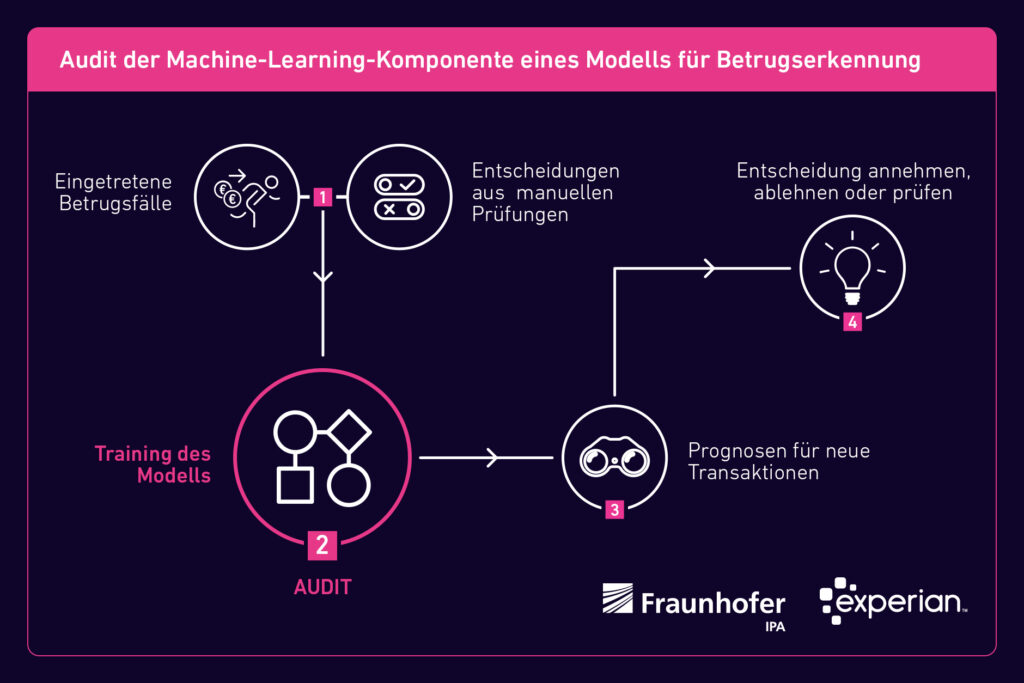
However, these methods are often insufficient in practice because they can only make statements for a specific model. However, in the case of a product like the Fraud Miner, different models are used depending on the customer. In addition, the models with the Fraud Miner must be regularly renewed to adapt them to the rapidly changing procedures of the fraudsters. The Fraud Miner audit therefore focused on four components that are the basis of the actual model development.
Component 1: Feature engineering
Feature engineering assessment primarily involves eliminating common sources of error. Common sources of error are target leaks or training data that are inadvertently present in the test set, or unclear feature transformations. With a target leak, information of the target variable is already available in the features: For example, the service life of a battery must be calculated in years, but the features actually have a service life of weeks. In this case, Fraunhofer IPA saw neither a risk of target leaks nor a risk of leaks of the training data into the test set. The feature transformations applied were also assessed as transparent.
Component 2: Model selection and training
As mentioned previously, the Fraud Miner regularly needs to select, train and calibrate an updated model to enable the system to reliably detect the latest fraud patterns. Originally, there were three models to choose from, the hyperparameters of which were determined by an evolutionary algorithm. Hyperparameters are the parameters of the model that are used to control the training algorithm and whose values, unlike other parameters, must be set prior to the actual model training. Hyperparameters can therefore be, for example, the number of layers in a neural network or the depth of a decision tree. At the end of the model selection, the hyperparameter setting that delivers the most accurate results is selected. The final model is then trained with the determined hyperparameters and calibrated.
When training and selecting models, it is important to ensure that current, well-known frameworks are used wherever possible and that optimisation takes place with respect to logical metrics. The use of such frameworks ensures the essential transparency and traceability in this step. In the case of the Fraud Miner, researchers found that all the methods used were established procedures and frameworks, apart from a sub-component developed specifically by Experian. Documentation and justifications for the choice of method are particularly important when implementing such methods. These were submitted in the audit.
Component 3: Model evaluation
After all training and calibration steps, a final evaluation of the finished model is performed on the test data set. At the end of a full training cycle, the “confusion matrix” is created for the respective classification problem. The false positive rate can, for example, be obtained from this, and a calibration report is also generated. This produces some key economic figures such as the markets and application scenarios of the respective customer with profit and loss accounts. The report is also important, because it takes into account fairness in the form of error rates for sensitive groups.
Component 4: Human control
The unease about AI and machine learning is also based on the idea that technical systems would continue to develop in permanent self-optimization loops. However, the experts at Fraunhofer IPA envisaged no problems in this case. Human experts and risk assessors are involved in model development and model evaluation and check the model for plausibility of expenditure. Similarly, the final decision always rests with a human being.
Conclusion
The Fraunhofer IPA confirmed the very high quality of the Fraud Miner during the entire development process and in the evaluation of transactions. The system makes reliable predictions that are comprehensible to experts and lead to improved decisions. No generally established standards currently exist to certify ML systems, the fairness and traceability of the Fraud Miner’s results cannot yet be officially certified. However, the audit comes as close as possible to a certification and has created the basis for the development of standardised audit procedures.
This type of project is part of the “reliable AI” research focus of the IPA’s Cyber Cognitive Intelligence (CCI) department, in which the researchers advise and support companies on AI developments and implement AI applications. An audit similar to that described can be implemented across all sectors for almost all AI-based applications.
“Machine learning gives us new tools at our disposal”
An interview with Martin Baumann, Director Analytics at Experian
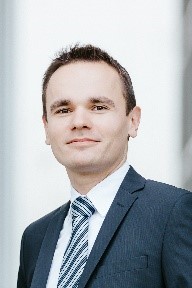
Mr. Baumann, what potential do you see in AI and more specifically machine learning in general and specifically for your company?
Even if we are still a long way from artificial intelligence as presented by Hollywood, the possibilities that AI and machine learning open up are already impressive today. Let’s think, for example, of talking devices or facial recognition on smartphones, as well as process automation in inboxes or the detection of anomalies in sensor technology. These applications have found their way into everyday life of private individuals and companies, and it is impossible to imagine life without them. Further digitization will lead to even more available data on which AI and ML are built. There are forecasts that attribute double-digit growth in global GDP by 2030 to the use of intelligent systems alone. For Experian, it is machine learning in particular that we are using to unlock new potential. Figuratively speaking, our data scientists have new tools at their disposal. This enables us to take into account additional types of data, for example, to design solutions that are even more tailored to individual customers, and to identify interdependencies that are more complex and map them in forecasting models. From an operational perspective, we can also work more efficiently. The Fraud Miner mentioned in the article is a good example of this.
How did you experience the audit process and what effort was involved?
The Fraunhofer IPA staff conducted the audit in a very professional manner. Despite the lack of precise specifications for the audit of an ML system, the merging of the three cited guides into an audit program was very smooth for us. The first step was to agree on the exact scope. After a general introduction to Experian and the use case of the Fraud Miner, there was a focus workshop on each of the four components, in which the model developers from Experian first presented the procedure to the Fraunhofer testers, in some cases down to code level, and then answered questions. If necessary, there was a second meeting or written exchange in which further queries were dealt with. Since this is a complex matter, we expected that there would be a considerable amount of work. Nevertheless, thanks to the structured approach, the number of appointments remained manageable. It should be emphasized that the atmosphere throughout had little of an examiner-examinee situation, but rather that discussions were conducted by experts with experts at eye level.
What lessons did you and your company learn?
The primary goal was the independent auditing of the Fraud Miner. Since we operate in the sensitive financial environment, it is our self-image that we must meet the highest standards. The review by a third party rounds off the many years of activity and high level of expertise of our colleagues. In the run-up to the audit, we were already convinced of the very high quality of the Fraud Miner. The confirmation in the audit result was nevertheless once again very pleasing. We will be happy to incorporate one or two suggestions from the expert discussions into our standards in the future. This is particularly true with regard to the secondary objective. It can be assumed that the ongoing legislative processes at European level, such as the EU AI Act mentioned, will generally bring new requirements for us as well as for many other companies. It is helpful to know that we are already well-positioned with our standards.
Mr. Baumann, thank you for this interview.
Contact
Prof. Dr.-Ing. Marco Huber
Head of the Department Cyber Cognitive Intelligence
Phone +49 711 970-1960